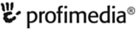
Profiset is a collection of 20M high-quality images with rich and systematic annotations, which were obtained from Profimedia, a web-site selling stock images produced by photographers from all over the world. For each image, we have extracted visual descriptors that can be used to search the images by content. Each entry in the dataset consists of the following information:
- a thumbnail image;
- a link to the corresponding page on the Profimedia web-site;
- two types of image annotation: a title (typically 3 to 10 words) and keywords (about 20 keywords per image in average) mostly in English (about 95 %);
- DeCAF7 descriptor extracted from the original image content using deep neural network;
- five MPEG-7 visual descriptors extracted from the original image content: Scalable Color, Color Structure, Color Layout, Edge Histogram and Region Shape.
To take a look at the collection, you can use the public demo and try our content-based searching in the images.

Profiset download
To download the Profiset data, you first need to confirm the Profiset usage agreement. Subsequently, we shall provide you with the access details.
If you download and use the Profiset collection for research purposes, please, reference the following paper:
- Budikova, P., Batko, M., and Zezula, P. (2011). Evaluation Platform for Content-based Image Retrieval Systems. In Proceedings of International Conference on Theory and Practice of Digital Libraries 2011, LNCS 6966, pages 130-142, Berlin: Springer. ISBN 978-3-642-24468-1.
If you use the DeCAF descriptors for efficiency evaluation, you can reference the following paper:
- Novak, D., Batko, M., and Zezula, P. (2015). Large-scale Image Retrieval using Neural Net Descriptors. In Proceedings of SIGIR ’15, pages 1039-1040, ACM New York, NY, USA. ISBN 978-1-4503-3621-5.
Profiset evaluation platform
Apart from the Profiset data itself, we also offer to share data that can be used for evaluation of large-scale image search systems. In particular, we provide a set of query topics, and a partial ground truth for each query topic. All this data is also included in the download package.
To enable efficient testing of search methods, we have defined a set of 100 query topics. For each of them, a semi-automatically collected ground truth data verified by users is available. Each query topic is formed by single query image and a few keywords (typically one or two). The topics were selected using the Profimedia search logs and several examples of queries that we know from experience to be either easy or difficult to process in content-based searching. We also checked that there are enough relevant results for each query on the dataset. The following categories are represented by the topics: activity (5 queries), animal (8), art (6), body part (5), building (3), event (3), food (8), man-made objects (16), nature (16), people (12), place (9), plant (2), speciï¬c building (4), vehicle (3).
A ground truth for a given query topic should in ideal case contain an indicator of relevance for each object in the dataset. However, creating such ground truth requires enormous amount of human labour. Therefore, we only provide a partial ground truth for each query. We employed a set of 140 different search methods exploiting different approaches (text-based search, content-based search, combination of both) to obtain a set of candidate objects. These were then manually evaluated by our judges (lab members). The judges were asked to mark each object as very good, acceptable, or irrelevant, which we transformed into relevance levels of 100 %, 50 % and 0 %, respectively. Each query-object pair was evaluated at least twice, the final relevance of a result object is computed as an average of the collected evaluations.